Analysis of fragmented piezometric levels records: the ARTE (Antecedent Recharge Temporal Effectiveness) approach
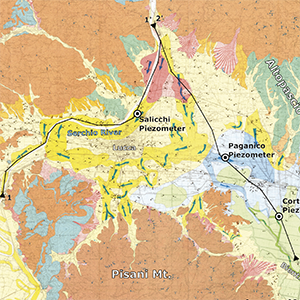
Submitted: 31 March 2022
Accepted: 21 June 2022
Published: 30 June 2022
Accepted: 21 June 2022
Abstract Views: 652
PDF: 445
Publisher's note
All claims expressed in this article are solely those of the authors and do not necessarily represent those of their affiliated organizations, or those of the publisher, the editors and the reviewers. Any product that may be evaluated in this article or claim that may be made by its manufacturer is not guaranteed or endorsed by the publisher.
All claims expressed in this article are solely those of the authors and do not necessarily represent those of their affiliated organizations, or those of the publisher, the editors and the reviewers. Any product that may be evaluated in this article or claim that may be made by its manufacturer is not guaranteed or endorsed by the publisher.
Similar Articles
- Francesca Lotti, Antonella Baiocchi, Salvatore D'Onofrio, Vincenzo Piscopo, Hydrogeological site characterization of marly-silici-calcareous rocks through surveys of discontinuities and pumping tests , Acque Sotterranee - Italian Journal of Groundwater: Vol. 1 No. 1 (2012)
- Giovanni Conte, Pio Di Manna, Rossella Maria Gafà, Lucio Martarelli, Gennaro Maria Monti, Marco Amanti, Sustainable yield of the Colle Quartara carbonate aquifer in the Southern Lepini Mountains (Central Italy) , Acque Sotterranee - Italian Journal of Groundwater: Vol. 5 No. 3 (2016)
- Lucia Mastrorillo, Roberto Mazza, Paola Tuccimei, Carlo Rosa, Renato Matteucci, Groundwater monitoring in the archaeological site of Ostia Antica (Rome, Italy): first results , Acque Sotterranee - Italian Journal of Groundwater: Vol. 5 No. 1 (2016)
- Lucio Martarelli, Marcello Iacuitto, Vincenzo Gregori, Riccardo Massimiliano Menotti, Marco Petitta, Anna Rosa Scalise, The Rieti Land Reclamation Authority relevance in the management of surface waters for the irrigation purposes of the Rieti Plain (Central Italy) , Acque Sotterranee - Italian Journal of Groundwater: Vol. 5 No. 2 (2016)
- Giuseppe Sappa, Flavia Ferranti, Francesco Maria De Filippi, A proposal of conceptual model for Pertuso Spring discharge evaluation in the Upper Valley of Aniene River , Acque Sotterranee - Italian Journal of Groundwater: Vol. 5 No. 3 (2016)
- Stefania Stevenazzi, Time-dependent methods to evaluate the effects of urban sprawl on groundwater quality: a synthesis , Acque Sotterranee - Italian Journal of Groundwater: Vol. 6 No. 4 (2017)
- Stefano Viaroli, Lucia Mastrorillo, Roberto Mazza, Vittorio Paolucci, Hydrostructural setting of Riardo Plain: effects on Ferrarelle mineral water type , Acque Sotterranee - Italian Journal of Groundwater: Vol. 5 No. 3 (2016)
- Maurizio Gorla, Roberto Simonetti, Chiara Righetti, Basin-scale hydrogeological, geophysical, geochemical and isotopic characterization: an essential tool for building a Decision Support System for the sustainable management of alluvial aquifer systems within the provinces of Milan and Monza-Brianza , Acque Sotterranee - Italian Journal of Groundwater: Vol. 5 No. 3 (2016)
- Behailu Birhanu, Seifu Kebede, Marco Masetti, Tenalem Ayenew, WEAP-MODFLOW dynamic modeling approach to evaluate surface water and groundwater supply sources of Addis Ababa city , Acque Sotterranee - Italian Journal of Groundwater: Vol. 7 No. 2 (2018)
- Halake Guyo Rendilicha, A review of groundwater vulnerability assessment in Kenya , Acque Sotterranee - Italian Journal of Groundwater: Vol. 7 No. 2 (2018)
You may also start an advanced similarity search for this article.